Paper delves into Privacy in Federated Learning
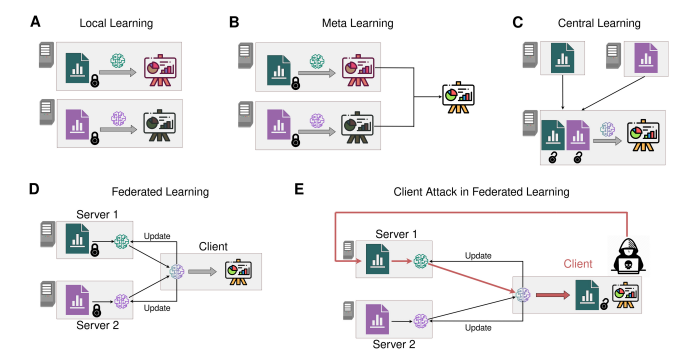
Researchers, including ORCHESTRA researchers from the University of Bonn and the Helmholtz Association, delved into enhancing the security of federated learning (FL). FL aims to allow for analyzing data in a privacy-safe manner while ensuring large sample sizes, hence it maintains high statistical power as a potent method for collaborative data analysis, especially in sensitive fields like healthcare. However, the risk of data leakage from malicious attacks is a critical concern. While already more secure than sending data around, attack algorithms could exploit information that is returned to the attacker in aggregated form.
Surprisingly, simple tools that are overlooked when screening a platform for possible vulnerabilities can lead to privacy breaches. The results show that Differential-Privacy can be a useful tool in order to prevent the types of attacks, which make use of basic descriptive statistics.
“Federated Learning is a great tool to ensure statistical power while upholding individual data privacy. However, it is essential that tool developers are aware of possible attack algorithms and pitfalls. When executed with precision and foresight, Federated Learning can significantly advance our comprehension of diseases through the lens of Machine Learning”, states one of the authors affiliated with the ORCHESTRA study.
Read the full article here: https://doi.org/10.1093/bioinformatics/btad531
###